Navigating Disaster Resilience: Flood and Tsunami Early Warning Systems with Machine Learning
Navigating Disaster Resilience: Flood and Tsunami Early Warning Systems with Machine Learning
(GF | 18/02/2025)
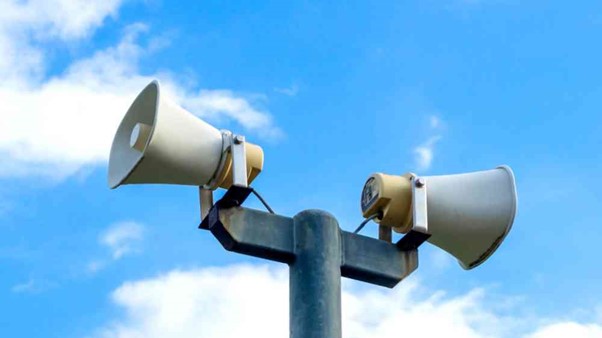
PT Bhumi Warih Geohydromatics supported by our expert, in collaboration with PT Telkom Indonesia and PT Metra-Net, has successfully developed an Early Warning System platform for floods and tsunamis in the Palu region. PT Bhumi Warih Geohydromatics was responsible for building both the backend and front end of the machine learning system and integrating it into the early warning infrastructure. This system is part of the Multi-Hazard Early Warning System (MHEWS), developed under the Indonesia Disaster Resilience Initiatives Project (IDRIP) initiated by the National Disaster Management Authority (BNPB). Using a machine learning-based approach, this system enhances disaster preparedness and response effectiveness, ensuring more efficient mitigation efforts.
The flood prediction system utilizes rainfall data as its primary input and employs a combination of Convolutional Neural Networks (CNN) and Long Short-Term Memory (LSTM) models. Meanwhile, the tsunami prediction model is based on earthquake parameters, using a CNN architecture. Prediction results are displayed in real-time through a web dashboard, providing crucial information such as warning status, estimated flood and tsunami inundation areas, tsunami arrival times, and the number of affected public and social facilities. Figures 1 to Figure 3 illustrate the web dashboard interface of the early warning system.
The application of machine learning in disaster early warning systems offers several key advantages:
- Time and Computational Efficiency: Compared to conventional numerical modeling methods, which require 3–6 hours, machine learning-based approaches can provide flood warnings within 15 minutes after rainfall data updates and tsunami warnings within 2 seconds after earthquake data updates.
- High-Spatial Resolution: Machine learning delivers detailed hazard predictions, with a spatial resolution of 5 meters for floods and 10 meters for tsunamis.
- Continuous Accuracy Improvement: The accuracy of the model increases as more high-quality training data is added, allowing the system to better recognize disaster-triggering patterns.
- Seamless Real-time Integration: Machine learning can be easily integrated into digital platforms, such as web dashboards, to disseminate information more quickly and widely to stakeholders.
The development of this system was made possible through the support of multiple stakeholders, including Indonesian Agency for Meteorological Climatological and Geophysics (BMKG) and the Ministry of Public Works and Housing (PUPR) as data providers. By integrating multiple data sources and leveraging expert knowledge, this system represents a significant advancement in technology-driven disaster mitigation in Indonesia. Building on its success, there is exciting potential for further development, with opportunities to enhance prediction accuracy, optimizing machine learning models, and enhancing computational capacity to enable wider implementation across different regions.
As a company specializing in hydrodynamics, meteorology, and climate, PT Bhumi Warih Geohydromatics remains committed to enhancing early warning system services to strengthen disaster resilience in Indonesia. Interested in discussing the implementation of this technology? Contact us via email at bw@bwgeohydromatics.com or connect with us on LinkedIn.